Artificial Intelligence (AI) Testing
Artificial Intelligence (AI) has experienced remarkable growth in recent years and has become a significant sector within the technology and business landscape. With this growth, software testing has become an important part of AI development for a number of reasons.
AI systems often rely on complex algorithms and models that require rigorous testing to ensure accuracy and reliability. Thorough testing helps identify and rectify any issues or biases in the AI system's decision-making process, ensuring that it performs as intended and provides reliable results. Testing can also help assess the robustness and generalisability of AI models by subjecting them to diverse datasets and scenarios, and uncovering potential limitations or vulnerabilities, enabling developers to refine and improve the system's performance.
Generally speaking, software testing for AI systems is vital to ensure their accuracy, reliability, fairness, interpretability, and security. By conducting rigorous testing, organisations can build robust and trustworthy AI systems that deliver optimal performance, comply with ethical standards, and provide value to users across various domains and industries.
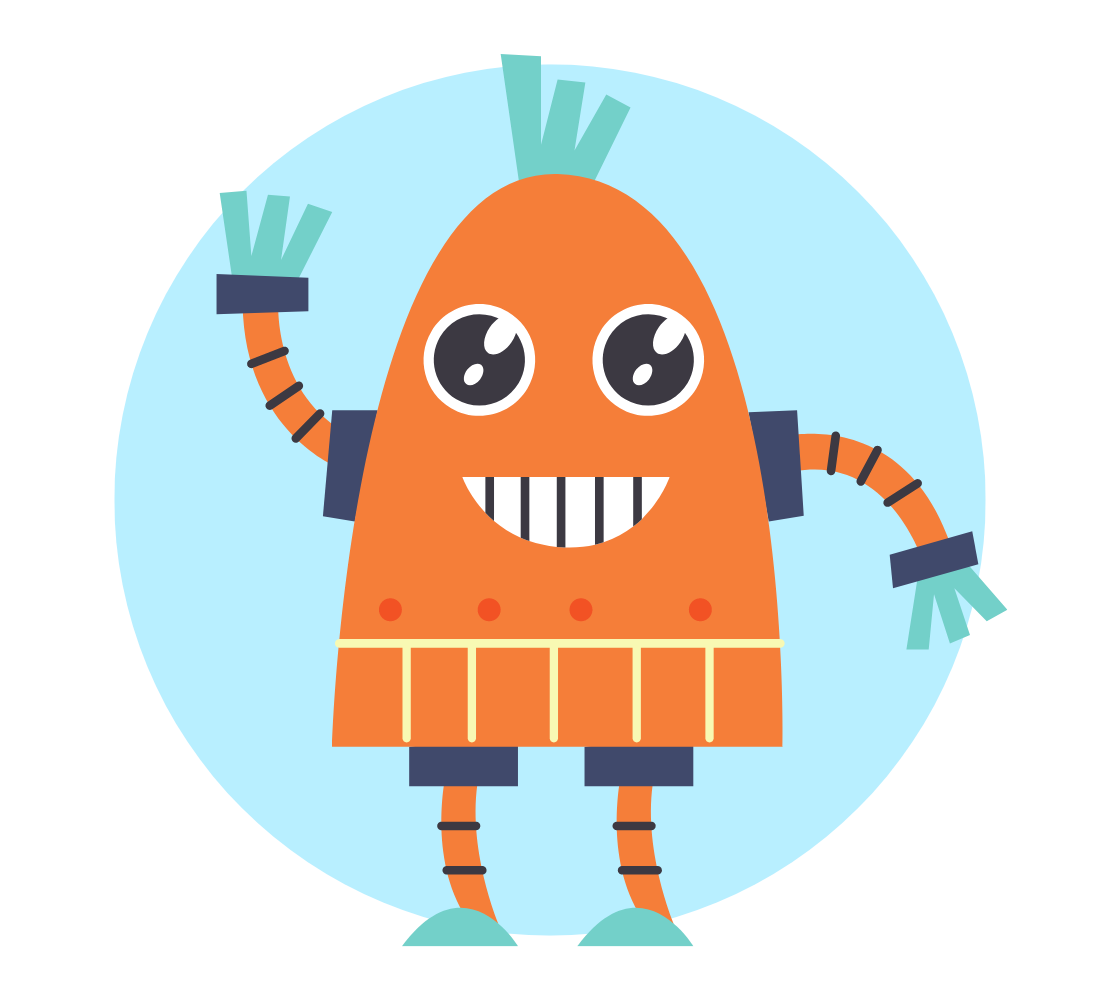
What are the top three considerations for AI Testing?

Data Quality
High-quality data is crucial for training and testing AI. Ensuring that the training data accurately represents real-world scenarios and covers a wide range of inputs helps improve the model's performance and generalisation capabilities. Data quality assessment, preprocessing, and validation techniques are essential to identify and address any issues in the training data.

Model Performance and Accuracy
Evaluating the performance and accuracy of AI models is vital. Testing should involve assessing the model's ability to make accurate predictions or decisions, measuring metrics such as precision, recall, F1 score, or accuracy.

Bias and Fairness
AI models are susceptible to biases that can lead to discriminatory outcomes. Testing should include assessing and mitigating biases in the model's training data and decision-making process. Evaluating metrics and conducting bias testing helps identify any biases that may result from the model's predictions or recommendations, ensuring equitable outcomes.
The first step is getting in touch
Message us at any time to tell us about your software or embedded software and one of our friendly team members will get back to you with further information.
We can set up a consultation either in person or online in order to discuss your specific requirements and for us to provide you with some initial recommendations.
Message UsEngagement and Process
After our initial consultation, you will be provided a scope of work and our formal Services Agreement.
Our scope of work will outline what testing methodologies are planned for your software (or the package you have opted for), the inclusions and exclusions, and is completely bespoke to your specific requirements.
Afterwards we will set up specified communication channels and work will begin.
View our Pricing GuideCommunication and Reporting
We aim to establish long-term partnerships built on the most appropriate structure to serve your requirements. Our team are seasoned communicators when it comes to testing and focus on client satisfaction, which means that you will be receiving ongoing productive feedback and suggestions.
At the end of our engagement, you will receive a formal Test Report. This report will outline any issues found within your software in a straightforward, efficient structure with only the necessary information.
Message Us